Anno Accademico 2022-2023
Vol. 67, n° 4, Ottobre - Dicembre 2023
Conferenza: Artificial Intelligence Applications in Oncology
16 maggio 2023
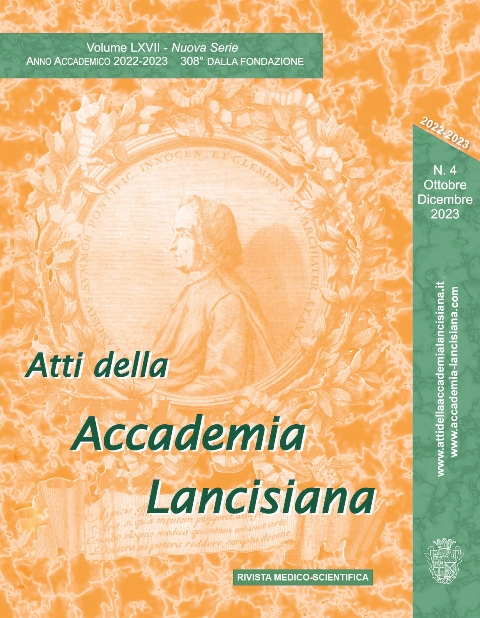
Conferenza: Artificial Intelligence Applications in Oncology
16 maggio 2023
Versione PDF dell'articolo: Download
The integration of artificial intelligence (AI) into everyday life has become an increasingly significant topic, presenting both promising advancements and potential drawbacks. AI has seamlessly integrated into our lives, as evidenced by voice assistants like Siri and Alexa. This article focuses on the concerning aspects of AI applications, particularly in the field of medicine, with a focus on oncology.
The term "artificial intelligence" originated around the mid-1950s and is defined by two characteristics: the ability to act humanly, like human beings, and the ability to act rationally. The first example of artificial intelligence is attributed to Alan Turing, renowned for the movie "The Imitation Game," which depicts the Enigma machine used by the Allies to decipher encrypted German messages during World War II.
Artificial Intelligence refers to the field of computer science and engineering that aims to create and develop systems, algorithms, and technologies capable of performing tasks that typically require human intelligence. These tasks encompass a wide range of cognitive functions, including reasoning, problem-solving, learning, perception, understanding natural language, and adapting to changing environments. AI systems often rely on techniques such as machine learning, neural networks, and pattern recognition to simulate and replicate human-like decision-making processes.
There are two crucial aspects of artificial intelligence. The first one is external, where we perceive intelligent behavior from the outside. The second one is much deeper, and this is perhaps the most significant aspect—the idea of a system that learns from data, acquires knowledge, and interprets it, progressively improving through internal circuits.
This year, the New England Journal of Medicine has dedicated articles and periodic issues specifically to artificial intelligence, underscoring its significance in the field. The reduction in the size of computer systems was associated with an increase in the speed of these devices. All of this has enabled science to perform calculations that were previously unthinkable, in a smaller space, and in a shorter amount of time, without the need for direct human involvement.
AI in General Practice
Artificial intelligence already plays a substantial role in public health and medicine, and its role is still evolving.
One example is its use in public health to identify outbreaks of infectious diseases, which can be calculated and detected by monitoring internet traffic or in hospital departments. The automatic interpretation of an electrocardiogram or a simple blood test also falls within the realm of artificial intelligence models that are still supervised by humans but represent excellent examples of its potential. These models can already lead to the identification of clinical trials simulated using artificial intelligence-controlled arms.
Furthermore, there is a wealth of data in Electronic Medical Records (EMR), which is already accessible on our mobile devices and can be transmitted. Artificial intelligence can also be employed in budget planning for individual companies or units or, as already done in the United States, in predicting insurance plans for patients.
Future developments may include chatbots like GPT-4, where artificial intelligence can assist a doctor during patient interviews and medical history taking. The interaction between the doctor and the patient is recorded and converted by AI into a medical visit, generating a report in medical language based on the patient's reported symptoms.
Another possibility is to use ChatGPT-4 as a help in teaching within a clinical setting. The chatbot not only provides the correct answer but also offers an explanation for why the answer is correct.
Artificial intelligence is a vast realm of algorithms designed to solve specific problems. These algorithms have evolved over time, giving rise to Machine Learning, Neural Networks, Deep Neural Networks, and Deep Learning1.
Machine Learning refers to a computer starting from experience, meaning a series of data, to respond to specific questions or tasks, which are assessed by performance indicators mentioned here. The performance of a particular task, measured through parameter P, improves with the number of experiences (E) related to some task class (T). The more data provided, the better the performance becomes2. Generally, the model is quite simple. A training set comprises initial data, following a paradigm completely different from the past. In the past, we had a hypothesis validated through observation, leading to a conclusion.
The critical transition between Machine Learning and Deep Learning lies in the inclusion of layered networks, known as Neural Networks or Convolutional Neural Networks (CNNs). These networks automatically re-analyze data, extract matrices, and uncover relationships between data points, enabling advanced analysis and accurate predictions.
This process is entirely inverted. It commences with a series of data, which are analyzed to various extents, and a theory is then formulated.
The pivotal step distinguishing Machine Learning from Deep Learning is the incorporation of these layered networks. They are referred to as Neural Networks, where the machine automatically reanalyzes the data and extracts matrices.
These systems are known as Convolutional Neural Networks. Thus, there is input of data, they are reanalyzed, reorganized, and spatially modified, providing a series of information ultimately leading to the final result.
All this internal component of the Neural Network is possible today due to significantly faster and more capable computer systems capable of analyzing this data and discovering relationships between data that we had not initially considered for analysis. Instances of Machine Learning can be found, for example, in protein configuration and spatial arrangement, with the precise identification of atoms in their correct positions, reaching up to 90%.
AI in Oncology
In the field of oncology, artificial intelligence has proven valuable in various areas, including cancer prevention, risk stratification, early diagnosis, assistive diagnosis, pathology, radiomics (the identification of specific radiological features for diagnosis), predictive outcomes, and teleoncology (remote cancer care)3.
1. AI – Assisted Diagnosis
AI can be used in radiomics to increase accuracy of lung cancer diagnosis from CT scans slides4.
A recently published study by Cheng et al., a PET-based radiomics machine learning model was employed to predict lymph node status in early-stage breast cancer. The AUC of the integrated model using radiomics models, clinicopathological data, ultrasound, physical examination, and lymph-PET as predictors was 0.94 (95% CI, 0.91-0.97, p<0.05) in the training set (n=203) and 0.93 (95% CI, 0.88-0.99, p<0.05) in the validation set (n=87), compared to 0.74 for lymph-PET in the training set or 0.765 for ultrasound in the validation set. In the N0 subgroup, the negative predictive value reached 96.88%, while in the N1 subgroup, the positive predictive value reached 92.73%. Cheng et al. demonstrated that the use of integrated models based on radiomics can improve the rates of true positives and true negatives for identifying clinical axillary lymph node status in early-stage breast cancer5.
2. AI – Identification of molecular profile from slides
Furthermore, Artificial Intelligence (AI) has progressed in predicting molecular status only based on tissue images, such as hematoxylin and eosin staining. One significant biological phenomenon considered is microsatellite instability, which affects DNA replication regulation, as observed in colorectal cancer (CRC) and Lynch Syndrome. Microsatellite instability, which disrupts DNA replication regulation, is observed in tumors like CRC, leading to mutations and base inversions during DNA replication. Four genes are involved in this process, which is crucial in the clinic, particularly in Lynch Syndrome—a genetically inherited condition associated with various cancers. Given that patients with Lynch Syndrome benefit from immunotherapy, its identification holds paramount importance in colorectal cancer patients.
AI's utility has been explored in molecular diagnostics to classify Microsatellite Stability (MMR) versus Microsatellite Instability (MSI), offering valuable insights for patient prognosis and treatment decisions. Dutch researchers from the National Cancer Institute in Amsterdam published a 2022 study wherein they applied the Deep Smile model, employing deep learning techniques, to predict homologous recombination deficiency (HRD) and microsatellite instability (MSI) in CRC and breast cancer. Through validation and training tests, the researchers analyzed histological preparations and successfully predicted MSI, achieving AUROC values ranging from 77% to 87% in colon cancer patients. In breast cancer patients, Deep Smile improved HRD classification performance from an AUROC of 0.77 to 0.816.
Ding et al. proposed graph neural networks for colon cancer, based on spatializing tumor tiles, to evaluate predicting cross-level molecular profiles of genetic mutations, copy number alterations, and functional protein expressions from whole-slide images. Through these deep learning systems, it is possible to spatially identify within the slide those patients who may exhibit alterations not only in DNA and RNA but also in the expression of individual proteins. Patients with PTEN inhibition typically experience a worse prognosis. In this study, the PTEN pathway, which is involved in signal transduction from epidermal growth factor receptors (EGFR), achieved an AUC of 0.867.
3. AI – Prediction of response
Another intriguing aspect is the use of AI to identify patients who may respond to immunotherapy. The prognosis of patients with metastatic non-small cell lung cancer has improved in recent years with anti-PDL-1 treatments. In 2019, Mok et al. demonstrated that patients with a PD-L1 expression level above 50% responded better to immunotherapy than chemotherapy, with a median OS of 20 vs 12.2 months8. Herbst et al.9 revealed that another anti-PD-L1, Atezolizumab, in patients with high PD-L1 expression, resulted in a better prognosis when treated with immunotherapy rather than chemotherapy. Immunotherapy has now become the preferred treatment for these specific patients.
A study published in Lancet Oncology in 2018 by Sun et al. aimed to develop and validate a radiomics-based biomarker capable of assessing the presence of tumor-infiltrating CD8 cells in patients receiving anti-PD-1 or anti-PD-L1 monotherapy in phase 1 studies. The researchers conducted a retrospective multicohort study using four independent cohorts of patients with advanced solid tumors. They combined contrast-enhanced CT images with RNA-sequencing genomic data from tumor biopsies to create a radiomic signature indicative of CD8 cell tumor infiltration. The radiomic signature was developed and validated in these cohorts, and its concordance was assessed in the Cancer Genome Atlas database. The radiomic signature's ability to predict the gene expression signature of CD8 cells in the TCGA validation set was shown to have an AUC of 0.67 (95% CI 0.57–0.77; p=0.0019). The radiomic score was significantly higher in the immune-inflamed group with dense CD8 infiltration (median 1.99, IQR 1.59–2.02) than in the immune-desert group with low CD8 cell infiltration (1.55, 1.50–1.75). In patients treated with immunotherapy, the radiomic score was also associated with a higher proportion of patients with an objective response rate at 3 (p=0.049) and 6 months (p=0.025), respectively, than those with progressive or stable disease. This imaging predictor shows great promise in predicting the immune phenotype of tumors and inferring clinical outcomes for cancer patients treated with anti-PD-1 and PD-L1 immunotherapy. The imaging biomarker has the potential to be valuable in estimating CD8 cell count and predicting the clinical outcomes of patients undergoing immunotherapy10.
In a study by Trebeschi, 1055 primary and metastatic lesions of 203 patients affected by melanoma and non-small cell lung cancer (NSCLC) treated with anti-PD-L1 were analyzed. An AI characterization of each lesion's contrast-enhanced CT imaging data was performed to develop and validate a noninvasive machine learning biomarker model capable of distinguishing between immunotherapy responders and non-responders. The biomarker showed significant performance in NSCLC lesions (up to 0.83 AUC, p<0.001) and borderline significance for melanoma lymph nodes (0.64 AUC, p=0.05). Combining the lesion-wide predictions on a patient level, they could predict immunotherapy response with an AUC of up to 0.76 (p < 0.001) for both cancer types, resulting in a 1-year survival difference of 24% (p < 0.02). These findings suggest that radiographic characteristics of lesions may become noninvasive biomarkers for immunotherapy response, also improving patient stratification for neoadjuvant and palliative treatments11.
In conclusion, artificial intelligence in oncology offers a significant opportunity to enhance diagnosis, predict molecular profiles, responses to treatments, and patient outcomes. However, challenges remain regarding data acquisition, standardization, algorithms, legislation, and ethics. Collaboration between clinicians and data analysts is pivotal to developing innovative and interpretable models that can integrate various data sources. The potential impact of artificial intelligence in improving patient care is enormous and will likely become an integral part of medical practice in the future.
BIBLIOGRAFIA